10 Data Science Use Cases in Insurance Industry In Karachi Pakistan Dubai
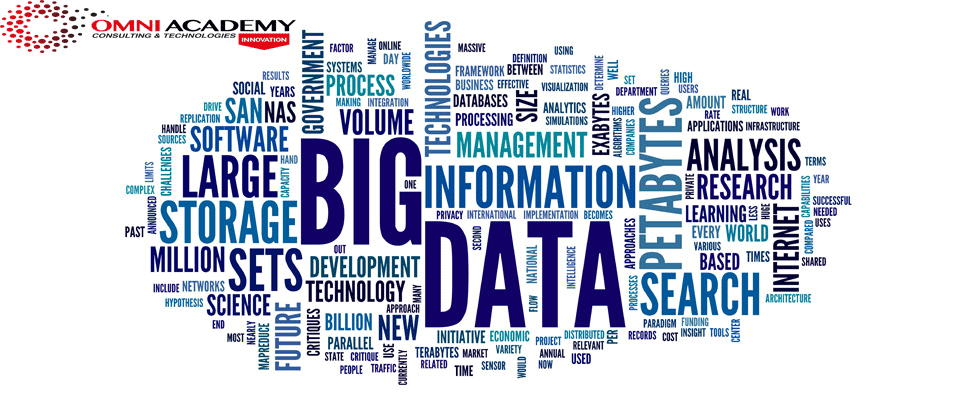
The insurance industry is regarded as one of the most competitive and less predictable business spheres. It is instantly related to risk. Therefore, it has always been dependent on statistics. Nowadays, data science has changed this dependence forever.
Now, insurance companies have a wider range of information sources for the relevant risk assessment. Big Data technologies are applied to predict risks and claims, to monitor and to analyze them in order to develop effective strategies for customers attraction and retention. Undoubtedly, the insurance companies benefit from data science application within the spheres of their great interest. Therefore, we have prepared the top 10 data science use cases in the insurance industry, which cover many various activities.
Fraud detection
Insurance fraud brings vast financial loss to insurance companies every year. Data science platforms and software made it possible to detect fraudulent activity, suspicious links, and subtle behavior patterns using multiple techniques.
To make this detection possible the algorithm should be fed with a constant flow of data. Usually, insurance companies use statistical models for efficient fraud detection. These models rely on the previous cases of fraudulent activity and apply sampling method to analyze them. In addition, predictive modeling techniques are applied here, for the analysis and filtering of fraud instances. Identifying links between suspicious activities helps to recognize fraud schemes that were not noticed before.
Price optimization
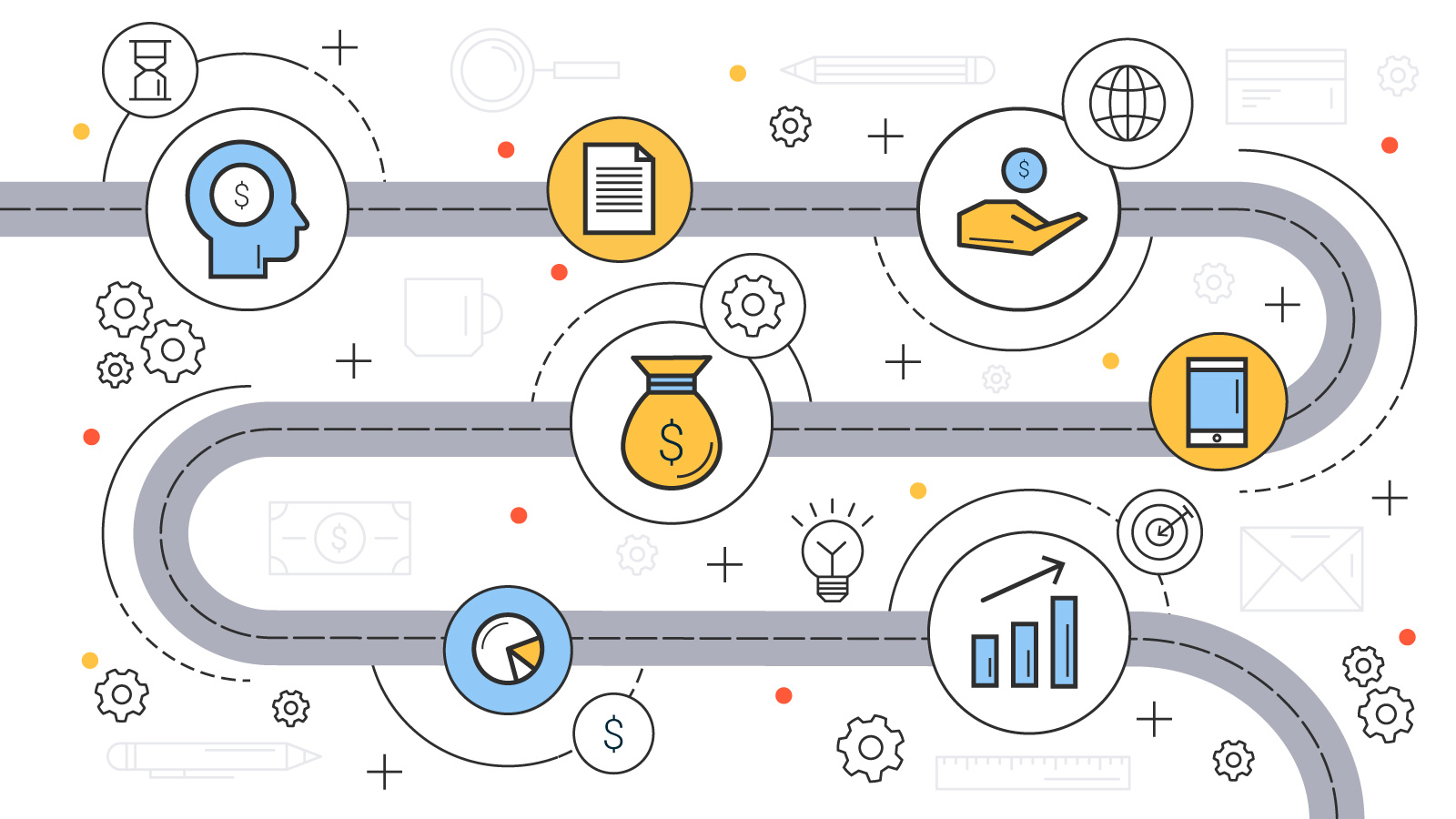
Price optimization procedure is a complex notion. Therefore it uses numerous combinations of various methods and algorithms. Despite the fact that it is still the disputable issue of applying this procedure for insurance, more and more insurance companies adopt this practice.
This process supposes combining the data not related to the expected costs and risk characteristics and the data not related to the expected loss and expenses, and its further analysis. That is, it takes into consideration the changes in comparison to the previous year and policy. Thus, price optimization is closely related to the customers’ price sensitivity.
In other words, historical costs, expenses, claims, risk, and profit are projected into the future. Special algorithms give the insurers the opportunity to adjust the quoted premiums dynamically.
As a key positive feature, price optimization helps to increase the customers’ loyalty in long perspective. Along with this, comes the maximization of profit and income.
Personalized marketing
The customers are always willing to get personalized services which would match their needs and lifestyle perfectly well. The insurance industry is not an exception in this case. The insurers face the challenge of assuring digital communication with their customers to meet these demands.
Highly personalized and relevant insurance experiences are assured with the help of the artificial intelligence and advanced analytics extracting the insights from a vast amount of the demographic data, preferences, interaction, behavior, attitude, lifestyle details, interests, hobbies, etc. The consumers tend to look for personalized offers, policies, loyalty programs, recommendations, and options.
The platforms collect all the possible data to define the major customers` requirements. After that, the hypothesis on what will work or won`t work is made. Here comes the turn to develop the suggestion or to choose the proper one to fit the specific customer, which can be achieved with the help of the selection and matching mechanisms.
The personalization of offers, policies, pricing, recommendations, and messages along with a constant loop of communication largely contribute to the rates of the insurance company.
Improve your skills with Data Science School
Customer segmentation
Modern technologies have brought the promotion of products and services to a qualitatively new level. Different customers tend to have specific expectations for the insurance business. Insurance marketing applies various techniques to increase the number of customers and to assure targeted marketing strategies. In this regard, customer segmentation proves to be a key method.
The algorithms perform customers’ segmentation according to their financial sophistication, age, location, etc. Thus, all the customers are classified into groups by spotting coincidences in their attitude, preferences, behavior, or personal information. This grouping allows developing attitude and solutions especially relevant for the particular customers.
As a result, target cross-selling policies may be developed and personal services may be tailored for each particular segment.
Lifetime value prediction
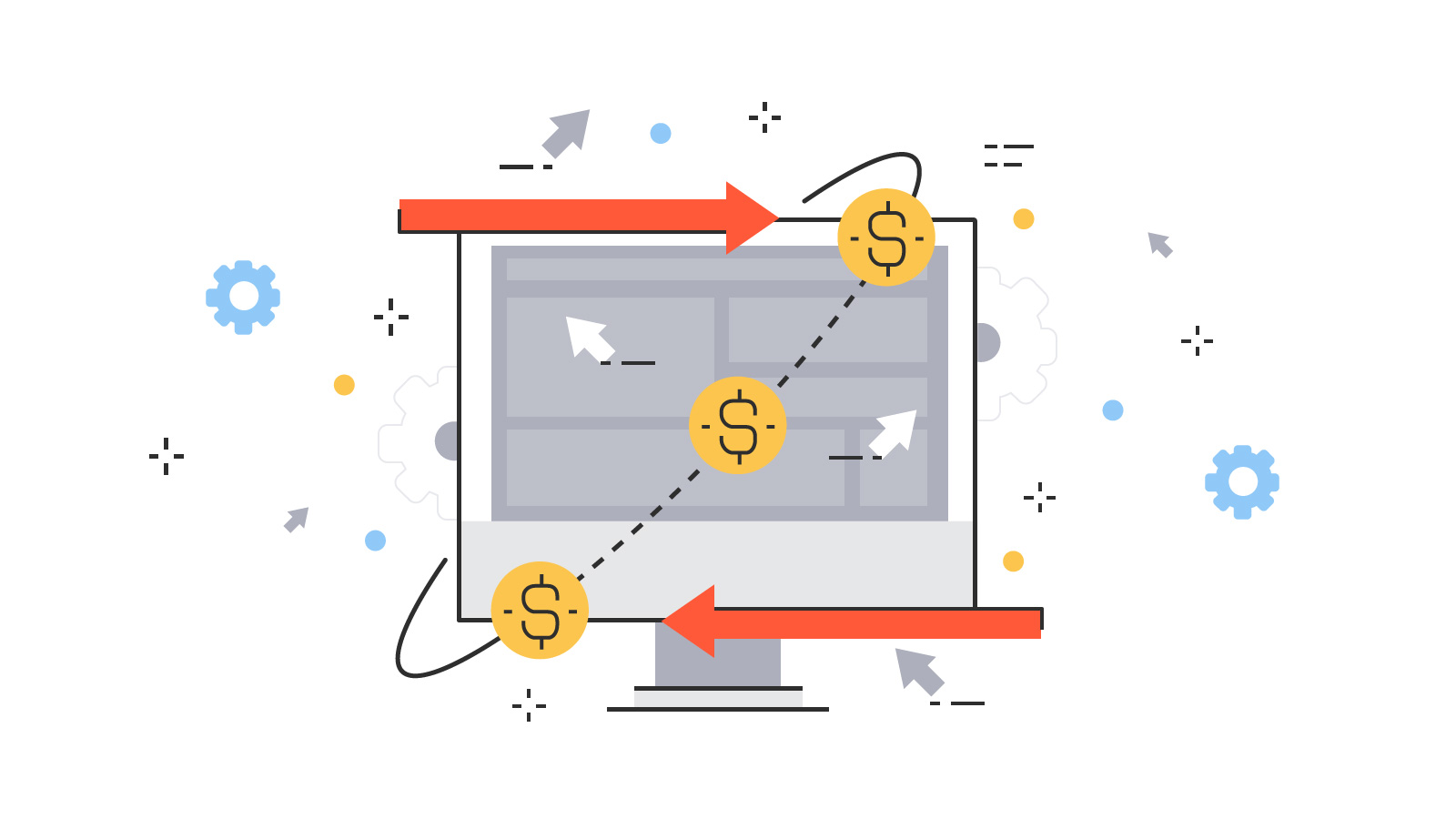
Customers lifetime value (CLV) is a complex phenomenon representing the value of a customer to a company in the form of the difference between the revenues gained and the expenses made projected into the entire future relationship with a customer.
Prediction of the CLV is typically assessed via customer behavior data in order to predict the customer’s profitability for the insurer. Thus, the behavior-based models are widely applied to forecast cross-buying and retention. Recency, a monetary value of a customer for a company and frequency are regarded as important factors to calculate future income. The algorithms put together and process all the data to build the prediction. This allows forecasting the likelihood of the customers’ behavior and attitude, maintenance of the policies or their surrender. In addition, the CLV prediction may be useful for the marketing strategy development, as it renders the customers’ insights at your disposal.
Recommendation engines
Recommendation engines are the algorithms applied to provide proper offers for each particular customer. They help to influence the customers’ day to day decisions, choices, and preferences.
These algorithms use special filtering systems to spot the preferences and peculiarities in the customers’ choices. The algorithms, also, include analysis of the data gained from simple questionnaires concerning demographic data and some personal information regarding the insurance experience and the insurance object.
On the basis of these insights, the engines generate more targeted insurance propositions tailored for specific customers. Thus, for example, the insurance company can avoid the ambiguity of the offering car insurance to a customer who is searching for a health insurance proposition.
Healthcare insurance
Healthcare insurance is a widespread phenomenon all over the world. It usually refers to the coverage of costs caused by the disease, accident, disability, or death. In many countries, the policies of healthcare insurance are strongly supported by the governments.
In the age of fast digital information flows this sphere cannot resist the influence of data analytics application. The global healthcare analytics market is constantly growing. The insurance companies suffer from constant pressure to provide better services and reduce their costs.
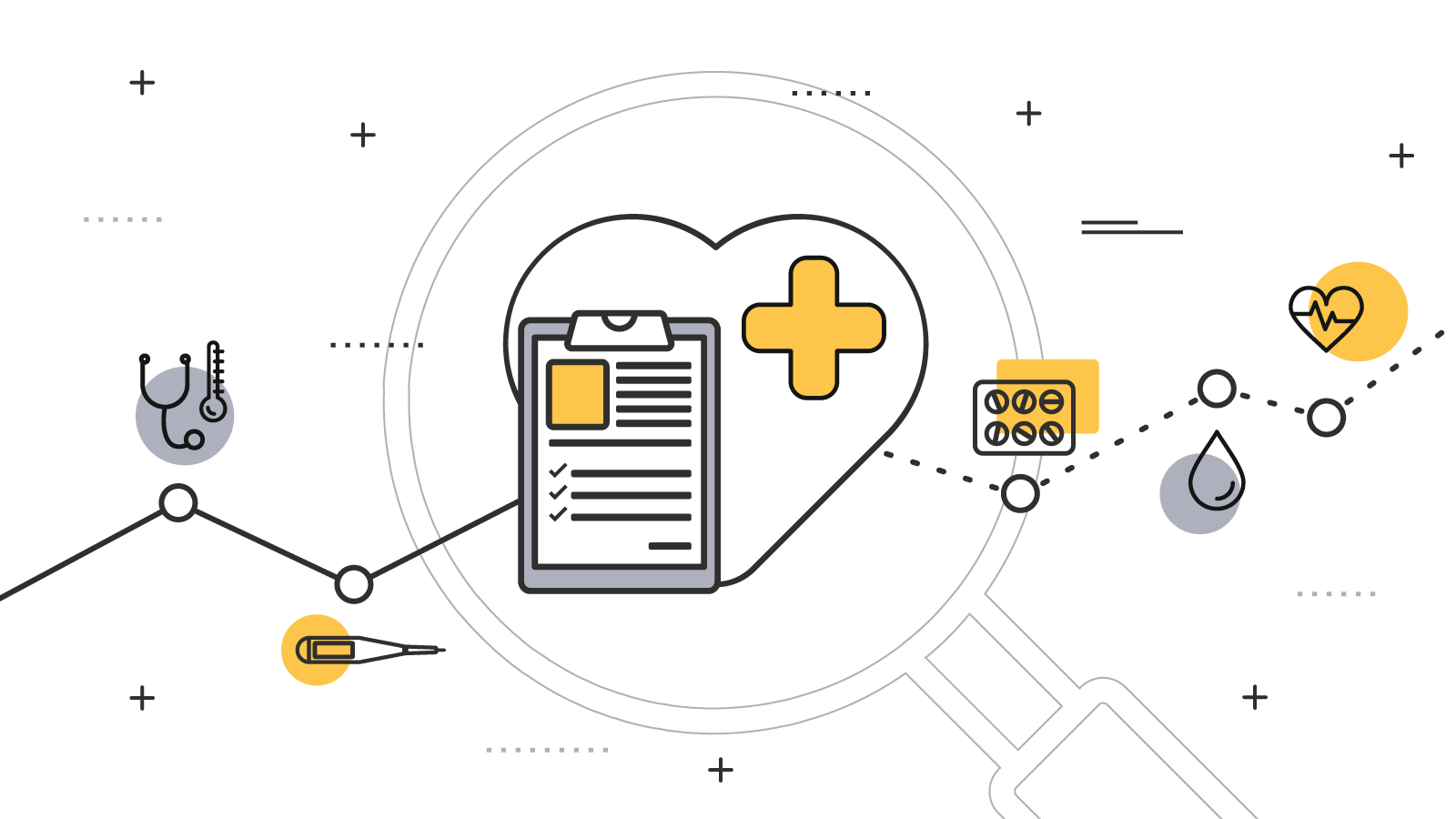
A wide range of data including insurance claims data, membership and provider data, benefits and medical records, customer and case data, internet data, etc. are gathered, structured, processed and turned into valuable insights for the healthcare insurance business. As a result, the aspects such as costs reduction, quality of care, fraud detection and prevention, and consumers engagement increase may be significantly improved.
Risk assessment
Implementation of the risk assessment tools in the insurance industry assures the prediction of risk and limits it to the minimum in order to cut losses. There are two major types of risk: pure and speculative. The risk assessment process is called to bring balance to the company’s profitability and to avoid both these types.
Risk assessment lies in identifying the risk quantification and the risk reasons. These are the basis for data analysis and calculations. The matrix model of the analysis is widely applied in this field. This model provides a systematic approach to risk information comparable in time. It is based on the algorithms which detect and combine the data concerning individual risks which vary by nature, character, and effect. Then, the potential risk groups are assessed. Thus, the overall company’s risk is forecasted via prediction of the exposure groups risks.
Claims prediction
The insurance companies are extremely interested in the prediction of the future. Accurate prediction gives a chance to reduce financial loss for the company.
The insurers use rather complex methodologies for this purpose. The major models are a decision tree, a random forest, a binary logistic regression, and a support vector machine. A great number of different variables are under analysis in this case. The algorithms involve detection of relations between claims, implementation of high dimensionality to reach all the levels, detection of the missing observations, etc. In this way, the individual customer’s portfolio is made.
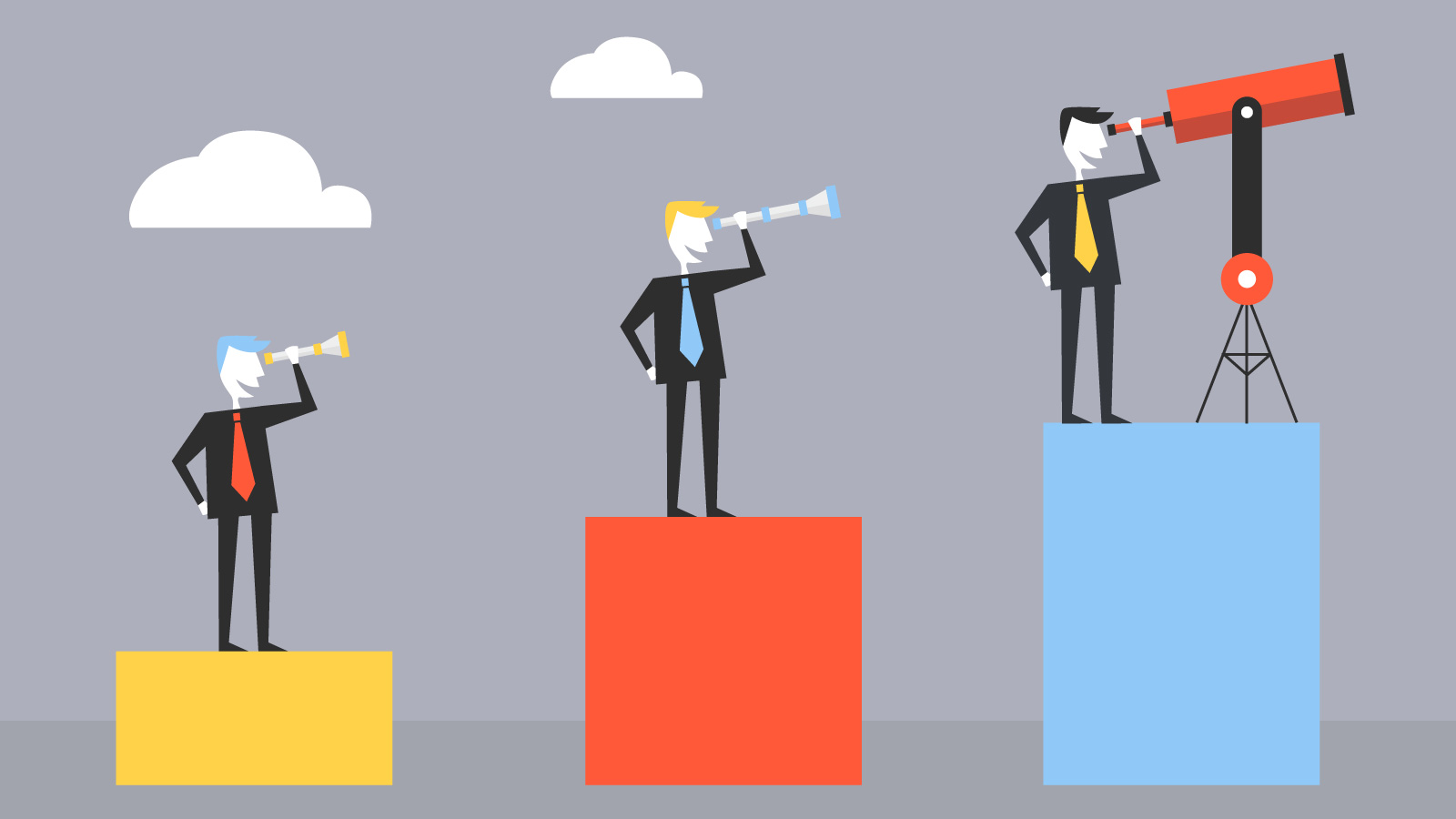
Forecasting the upcoming claims helps to charge competitive premiums that are not too high and not too low. It also contributes to the improvement of the pricing models. This helps the insurance company to be one step ahead of its competitors.
Automating life-event marketing
Under conditions of the highly-competitive insurance market, the insurance companies face the everyday struggle to attract as many customers as possible via multiple channels. Thus, the companies need to use comprehensive marketing strategies to achieve their goals. The automated marketing reaches its peak in this respect.
The automated marketing is a key to revealing the insights of the customers` attitude and behavior via initial research, product inquiry, purchases, and claims. As the main goal of digital marketing is to reach a right person at a right time with a right message, life-event marketing is more about the special occasion in the customers’ lives. Due to data science techniques, the insurers can collect the data from multiple channels and detect special dates and celebrations. Then, via complex algorithms and associations, targeted suggestions and strategies are applied.
Tracking the customer moving through the life cycle, the insurance companies guarantee themselves a constant flow of clients matching a wide range of their suggestions.
Conclusions
Modern technologies are moving extremely fast making their ways into various fields of the business. In this respects, the insurance industry does not lack behind the others. The application of statistics in the insurance has a long history. Thus, the fact that insurance companies are actively using data science analytics is not surprising.
In essence, the aim of applying data science analytics in the insurance is the same as in the other industries – to optimize marketing strategies, to improve the business, to enhance the income, and to reduce costs. In this article, we presented the most vivid examples of using the analytics tools and algorithms in the insurance industry to successfully achieve this aim.
Comments
Post a Comment